Artificial intelligence (AI). Generative AI. It’s a whole new world of possibilities. From powering personalized recommendations to enabling mass customization, generative AI’s influence already permeates nearly every facet of modern life.
But a pressing concern looms: ensuring that the data feeding these intelligent systems is relevant and reliable — and responsibly managed. This is where data governance plays a pivotal role.
In this article, I cover some core concepts defining AI and trace its development to today’s generative AI capabilities. Having a firm grasp of the subtleties between these two concepts, AI and generative AI, is critical to developing effective data governance strategies that will enable these solutions to deliver on their intended business value.
In future articles on FSFP’s blog, we’ll explore generative AI more deeply, examining business and data governance approaches to get the most from this new technology.
What Is AI?
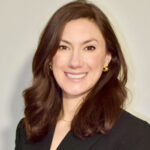
I was one of FSFP’s presenters at the March 2024 Enterprise Data World event. My session, Generative AI for Data Governance and Business Transformation, generated some great questions and ideas for future blog articles … so stay tuned!
In the public imagination, the term AI evokes a sense of modernity and progressiveness that we’ve just begun to explore. However, for those in more technical fields, the concept of AI is not new. Its roots date back several decades, with initial research, discussions and models emerging as early as the mid-20th century.
One way of defining AI is as a field of study within the computer science discipline dedicated to the simulation of human intelligence by machines.
Early AI systems were developed to perform a specific task — from playing checkers to solving algebra word problems. As technology has progressed and computing power has increased, the types of tasks an AI system is programmed for have also increased in sophistication and complexity. Instead of playing checkers, AI is now playing chess. Instead of solving specific algebra problems, it now solves general knowledge problems on Jeopardy.
What Is Machine Learning?
Machine Learning (ML) is a term we often hear alongside (or in place of) AI. Until recently, ML was the primary subfield of AI where development and business application took place.
ML is a system that is programmed to perform a specific task, leveraging supervised learning techniques. Supervised learning requires the labeling of input data sets and the acceptance/rejection of outputs. As a result, the input data is mainly in a structured or semi-structured format so it can be annotated (labeled) by engineers or data stewards.
While ML developed in its sophistication, technologies continued getting faster and more powerful. Simultaneously, the amassing of digital data was beginning to hit critical mass levels. These factors have ushered in a new field of AI called deep learning.
What Is Deep Learning?
Deep learning is the new frontier of AI. It’s the field where recent advancements have exploded onto the scene — namely, generative AI.
Deep learning is a subfield of ML wherein machines can learn from previous experiences to improve on their outputs. Deep learning leverages supervised learning as its training method. It automates the “labeling” of input data sets by self-identifying these features and doesn’t require human intervention to accept or reject the outputs. The reason for this is the sophistication and power of its underlying technology and the scale of data it can consume.
Neural networks are the technological backbone of how deep learning models work. This layered structure means feature extraction can be automated but vast amounts of data are needed for more accurate results. Since no human-driven labeling is required, deep learning models can consume structured, semi-structured and unstructured data.
Generative AI is a form of deep learning that uses data from all different formats (often called modalities) to create something new. We’ll explore generative AI in more detail in future articles on FSFP’s blog. (To make sure you get the series, sign up here.)
Functional Areas of AI
There are many ways of interpreting and classifying AI, but here are four key functional areas. We think of these as “horizons of innovation” where each phase builds in sophistication from the last:
- Reactive Machines – These machines operate without memory and are task-specific. ML models fall into this category — they have been programmed to do a certain thing. Recommendation engines and customer lifetime value models are examples of this.
- Limited Memory – Limited memory encompasses deep learning and reinforcement learning. With limited memory, its goal is to mimic human ability to learn through “experiences.” This means that as these models continue to be trained, they improve their results.
- Theory of Mind – This concept refers to imbuing machines with social intelligence. It involves understanding human emotions, intentions and interactions. As AI evolves, an emerging use case will be integrating machines into team environments.
- Self-Awareness – Self-awareness aims to give machines a sense of consciousness. (Some call this artificial consciousness or machine consciousness). While not yet realized, researchers and technologists actively explore this concept and the applications to support it.
Criticality of Data for AI
It may be an obvious statement, but it’s profound: AI needs data to operate. But it doesn’t just need any data, it needs trusted data — data that is secure, compliant and fit for purpose. Trusted data is the result of effective data governance. Whether it’s traditional machine learning models or generative AI, data is the true enabling (or limiting) factor for the accuracy of your AI models. But data governance’s role doesn’t end here. It’s our responsibility to keep a line-of-sight on the data throughout model development and ongoing deployment.
Up Next in Our AI Article Series
In future articles, we’ll continue laying the foundation for a business understanding of generative AI, its value and limitations. This foundation will explain how, when and where data governance can deliver the ultimate need for AI — trusted data.
How Can We Support Your AI Efforts?
At FSFP, we understand that successful AI implementations rely on a solid data governance foundation. Our 17 years of experience in data governance and management consulting, partnering with hundreds of clients nationwide, uniquely positions us to guide you through AI’s complexities.
Let’s unlock the full potential of AI while safeguarding one of your organization’s most valuable assets — your data. Get in touch with us to share what you’re working on and how we can assist you.